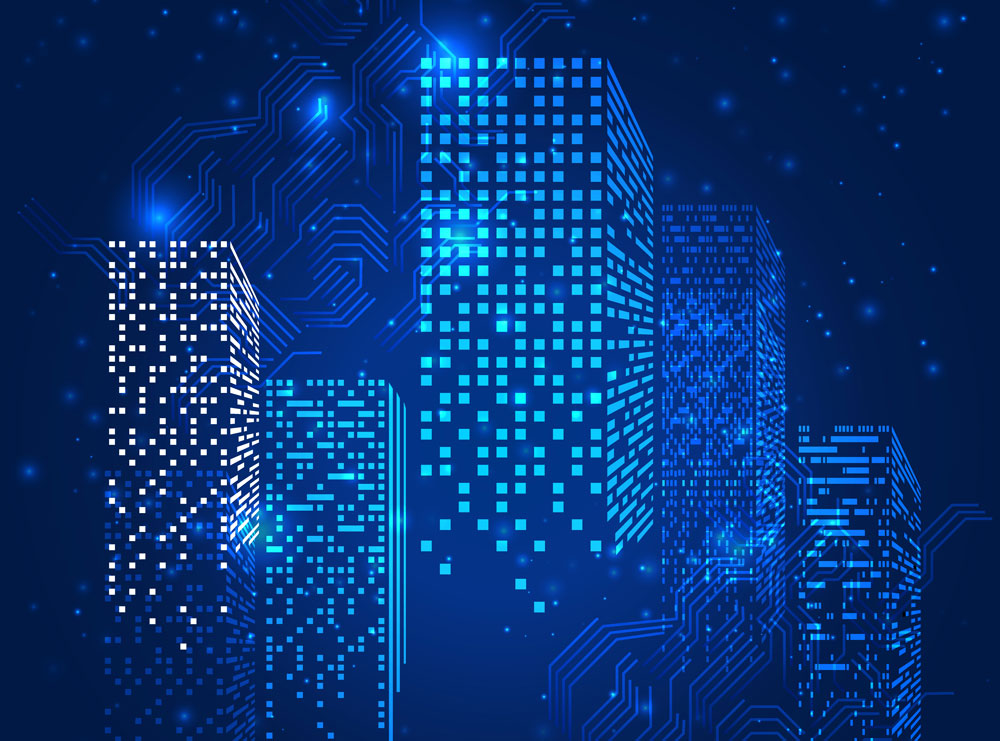
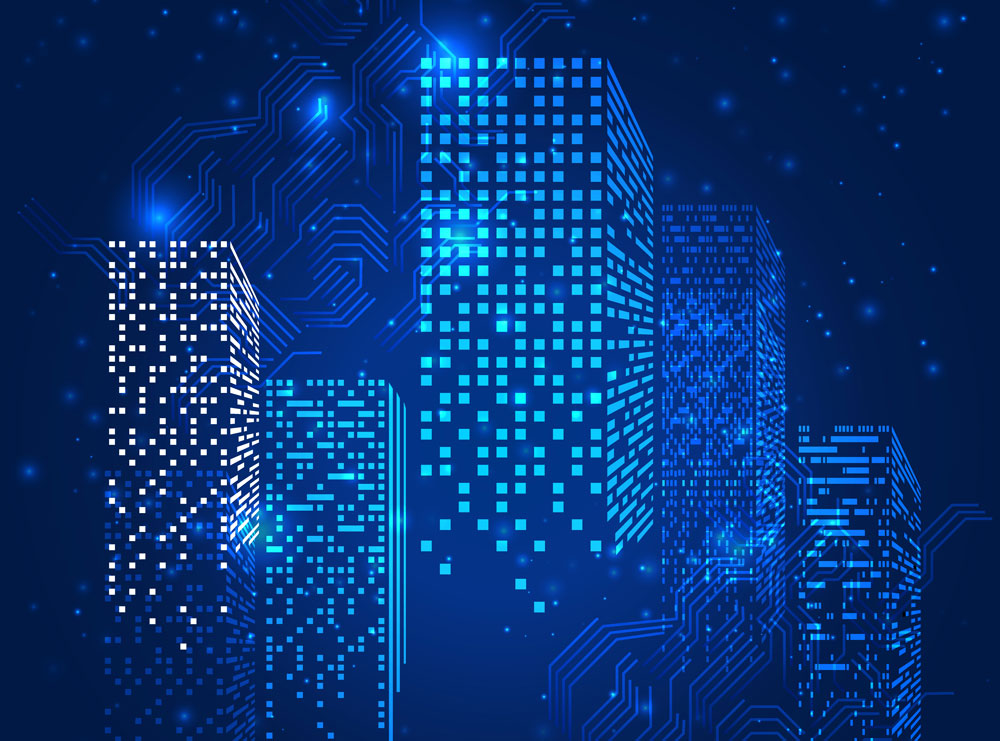
The use of data analysis methodologies in the field of energy intelligence has been developing significantly in the last years, leading to the definition of consolidated modeling approaches for data monitoring and processing. Such evolution was supported by the growth of the scientific knowledge in the field of Big Data Analysis, by the continuously increasing amount of data available and by the rise of the number of systems involved and their complexity.
The complexity of the systems analyzed and the experience acquired in field since the time of its foundation, has revealed the need for Ouvert to constantly use cutting-edge modeling approaches, keeping up with the evolution of algorithms in the field of Machine Learning.
Data clustering is one powerful feature used in these machine learning models: it allows for organizing and classifying data for a characterization of the analyzed systems, enabling the detection and mining of important information, such as:
- identification of patterns in time series acquired from meters
- classification of an energy system according to its features.
Combined with regression analysis, it allows to create dynamic forecast models, which are at the basis of all the energy intelligence functionalities provided by Ouvert’s solutions.
The great advantage in using Machine Learning algorithms is related to the capability they have to use the already processed data to improve the interpretation of subsequent data, following a cognitive evolution which is context-dependent. The self-learning features determine a continuous transformation of the models, with a real time adaptation to the information contained in the data, enabling the possibility to develop effective automation algorithms, like the ones implemented in Ouvert’s solutions.
These models can be applied to extremely complex networks of infrastructures to reach different objectives:
- support to decision-making when evaluating energy conservation measures
- effective design of monitoring networks
- analyze the efficiency of distributed systems making use of benchmark analysis
- enable prediction and detection of relevant events.
In case of benchmark analysis the reliability of the results has improved over the years thanks to the capacity of the models to better and better discriminate all the important benchmarking features, leading to a continuous improvement of the forecasting capability of the model.
In the same way, the analysis of historical time series has strengthened the capability of the models to identify important events, extract relevant time patterns and define actions, making the models more and more reliable, especially in case of recurrent contexts.
The use of Machine Learning algorithms is a crucial aspect in Ouvert’s approach to energy intelligence: the focus on mining complex relationships from the data gives an essential support to the execution of a project in all its phases: from design to data analysis to the evaluation of the efficiency goals.